BackTable / VI / Podcast / Episode #251
Race and AI in Radiology
with Dr. Judy Gichoya
In this episode, Dr. Ally Baheti interviews interventional radiologist Dr. Judy Gichoya about her recent paper on artificial intelligence (AI) and the use of a deep learning model to recognize patients’ self-described racial identity, based on radiology images.
This podcast is supported by:
Be part of the conversation. Put your sponsored messaging on this episode. Learn how.
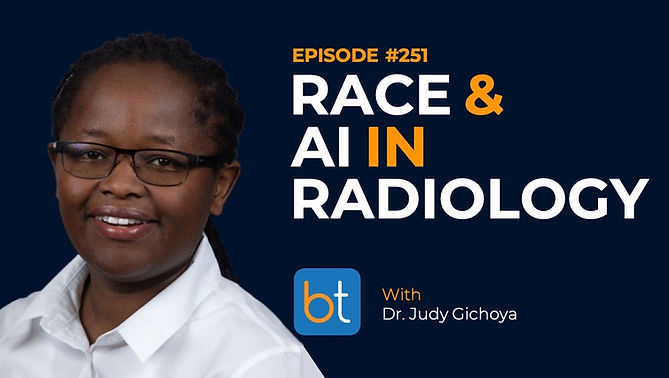
BackTable, LLC (Producer). (2022, October 14). Ep. 251 – Race and AI in Radiology [Audio podcast]. Retrieved from https://www.backtable.com
Stay Up To Date
Follow:
Subscribe:
Sign Up:
Podcast Contributors
Dr. Judy Gichoya
Dr. Judy Gichoya is an interventional radiologist, researcher, and assistant professor at Emory University School of Medicine.
Dr. Aparna Baheti
Dr. Aparna Baheti is a practicing Interventional Radiologist in Tacoma, Washington.
Synopsis
Dr. Gichoya had started by tackling the original problem of bias in diagnoses for chest X-rays, since it has always been difficult to tell whether something is a real diagnosis, or simply just a finding. Her team built a deep learning model; however, they saw that it did not work well for black patients. With further investigation, they discovered that their model had learned signals that correlated with self-identified race.
Intrigued by this finding, Dr. Gichoya and her team sought to identify the factors that the model used when making its race determination. Because AI is black box in nature, the methods by which the algorithm learns remains largely unknown. When tested in other imaging modalities (mammogram, chest CT, spine imaging), the model still showed high accuracy. Additionally, the model retained accuracy when different information was eliminated from the images (ex. age, disease distributions, bone densities). The model was also able to predict race in healthy patients, showing that it did not rely on patterns of disease prevalence in specific ethnic groups.
Next, we spoke about the implications of this research in developing risk scores. Deep learning models are able to look at factors that humans are not trained or able to see. Dr. Gichoya highlights the model’s potential effectiveness in predicting osteoarthritis risk in black patients. We also look at applications in opportunistic screening and information about social determinants of health. For example, most patients presenting with chest pain often get chest CTs. Dr. Gichoya thinks that these images can be used by the model to learn about patients’ environmental exposures, like pollution.
We finish the episode with a discussion on the changing landscape of IR and how AI can be used as an assistive technology. Interventional cardiologists are already using AI to dictate their procedural reports in real-time. In the interventional oncology space, AI could help integrate imaging and pathology findings to determine personalized treatment courses. All of these applications depend on researchers’ ability to market their findings to peers and the public, Dr. Gichoya gives tips on how to do this.
Resources
AI recognition of patient race in medical imaging: a modelling study:
https://www.thelancet.com/journals/landig/article/PIIS2589-7500(22)00063-2/fulltext
Disclaimer: The Materials available on BackTable.com are for informational and educational purposes only and are not a substitute for the professional judgment of a healthcare professional in diagnosing and treating patients. The opinions expressed by participants of the BackTable Podcast belong solely to the participants, and do not necessarily reflect the views of BackTable.